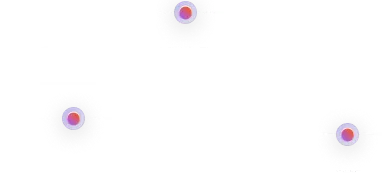
.png)

AI has changed how content is created, giving marketers new tools to make engaging material quickly. But as AI becomes more important in content creation, it's crucial to deal with bias in AI-generated content. Bias can affect how effective and fair AI outputs are, making it a big concern for marketers.
Bias in AI-generated content means the material produced by AI systems has unfair tendencies. This bias often comes from the data used to train these models, which can reflect existing social, cultural, or institutional biases. For marketers, understanding and reducing this bias is not just a technical challenge but also an ethical responsibility. Addressing bias ensures that content is inclusive, fair, and accurately connects with diverse audiences.
Tackling bias in AI-generated content is very important. For marketers, biased content can lead to misrepresentation, alienation of certain groups, and potential damage to their reputation. By actively working to reduce bias, marketers can create more fair content that matches their brand values and builds trust with their audiences.
Bias in AI systems shows up in different ways, often starting from the data used to train these models. According to DEPT®, "Bias in AI content can occur in a number of ways, but it typically stems from biases in the data used to train AI models." This means that if the training data contains biases related to race, gender, religion, or other characteristics, the AI model is likely to copy and even increase these biases in its outputs.
Selection bias is a common type of bias in AI systems. It happens when the data used to train the model doesn't represent the broader population. For example, if an AI model is trained mostly on data from a specific group, its outputs may favor that group, leading to skewed results.
The role of training data is crucial in shaping AI behavior. As highlighted by Google AI, "ML models learn from existing data collected from the real world, and so a model may learn or even amplify problematic pre-existing biases in the data based on race, gender, religion or other characteristics." This shows the need for diverse and representative data sets to train AI models, ensuring that the AI outputs are fair and unbiased.
Understanding these concepts is the first step towards addressing bias in AI-generated content. By recognizing the sources and signs of bias, marketers can better plan how to reduce these issues and produce more ethical and inclusive content.
The data used to train AI models is very important in shaping their behavior. As highlighted by Google AI, machine learning models learn from existing data collected from the real world. This means that if the training data contains biases, the AI model may replicate these biases and even make them worse. For example, if the data reflects gender stereotypes, the AI-generated content might continue these stereotypes, leading to biased outcomes.
Understanding these aspects of bias in AI is important for marketers. By recognizing how bias can enter AI systems, they can take steps to ensure their content is fair and inclusive. This involves using diverse and representative data sets and continuously checking AI outputs for signs of bias.
Selection bias happens when the data used to train an AI model doesn't represent the broader population. For example, if an AI model is trained mostly on data from a specific demographic, its outputs may favor that group too much. This can lead to skewed results and unfair advantages or disadvantages for certain parts of the population.
Algorithm bias happens when an AI system consistently favors one group over another based on things like race, gender, or socioeconomic status. This bias can come from the design of the algorithm and the data it uses. For instance, an AI algorithm used in hiring might favor candidates from certain backgrounds if the training data reflects past hiring biases. As noted by Aicontentfy, such biases can keep inequality going and make AI-driven systems unfair.
Machine learning bias refers to the systematic error introduced by an AI model due to biased training data. This bias can affect the model's predictions and decisions, leading to skewed outcomes. For example, if a machine learning model used for credit scoring is trained on data that reflects historical discrimination, it might unfairly penalize certain demographics. As highlighted by HBR, AI-driven systems are subject to the biases of their human creators, making it essential to address these issues at the source.
Bias in AI-generated images can be sneaky because it often goes unnoticed by most people. These biases come from the datasets used to train the AI, which might not show the real world's diversity. For example, if an AI model is mostly trained on images of a specific race or gender, it might produce outputs that favor those groups, leaving others out. This can have big effects, especially in advertising, where visual content shapes public perception.
Generative AI, which creates new content based on existing data, is especially prone to bias. This is because the AI learns patterns from the training data, which may have hidden biases. For example, if generative AI is used to create marketing copy, it might accidentally reinforce stereotypes if the training data includes biased language. This can result in content that is unfair and potentially harmful to certain groups.
Real-world examples of AI bias are everywhere. For instance, facial recognition technology has been shown to have higher error rates for people of color compared to white individuals. This happens because of the lack of diversity in the training datasets. Another example is the use of AI in predictive policing, which has been criticized for disproportionately targeting minority communities. These examples show the urgent need to address bias in AI-generated content to ensure fairness and equity in AI applications.
Reducing bias in AI systems needs several approaches. One effective method is feature blinding, which means removing or hiding sensitive attributes from the data before training AI models. This helps ensure the AI doesn't make decisions based on biased information. Another technique is adversarial classification, where models are trained to spot and reduce bias actively. Also, changing the objective function to focus on fairness over accuracy can lead to fairer outcomes. These methods, among others, are important for making AI systems fairer (TechTarget).
Human psychology plays a big role in AI bias. Our natural biases can slip into the data we collect and the algorithms we design. For example, confirmation bias might make developers favor data that supports their preconceptions. Understanding these psychological factors is key to creating unbiased AI systems. By being aware of our biases, we can take steps to counteract them, like including diverse perspectives in the development process and continuously learning about the impact of bias.
Curating diverse and representative datasets is crucial for reducing bias in AI-generated content. Ensuring that training samples include a wide range of backgrounds, genders, and experiences can help create more balanced AI models. This means actively seeking out and including data from underrepresented groups to avoid skewed results (DEPT®).
Human oversight is key to ensuring ethical AI content. Regularly reviewing and auditing AI outputs can help identify and fix biases that may have been missed during development. This process involves not just technical experts but also ethicists and representatives from diverse communities to provide a thorough review (Agency Partner).
The future of AI-generated content will likely see continuous improvements in fairness and transparency. By investing in ongoing education and adapting to new ethical guidelines, we can ensure that AI systems become more equitable. Regularly monitoring AI for biased outcomes and adjusting models as needed will be essential to maintaining fairness over time (The Uncommon League).